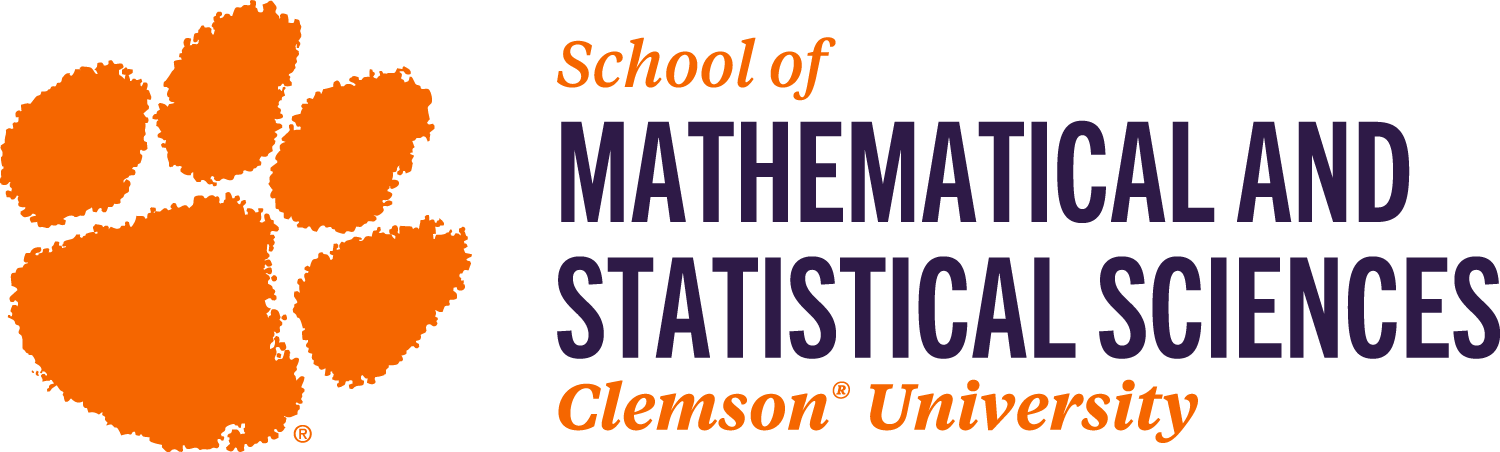
More Than Number-Crunchers
As a graduate from the Clemson University School of Mathematical and Statistical Sciences, you will be prepared to enter the job market as a problem-solver who has received broad instruction to prepare you for an exciting and rewarding career in an almost-limitless range of fields.
Modern mathematicians do more than crunch numbers. As a Clemson-trained mathematician, you will be a problem solver who enters the workforce with all the tools you need to provide valuable contributions that help improve the quality of life for everyone in myriad ways. You will also be trained to communicate your solutions clearly and logically to others. Whether you pursue a career in industry, health care, life sciences or other field, or opt to dedicate yourself to academics and pure research, a degree in mathematical and statistical sciences provides a pathway to a successful, rewarding career.
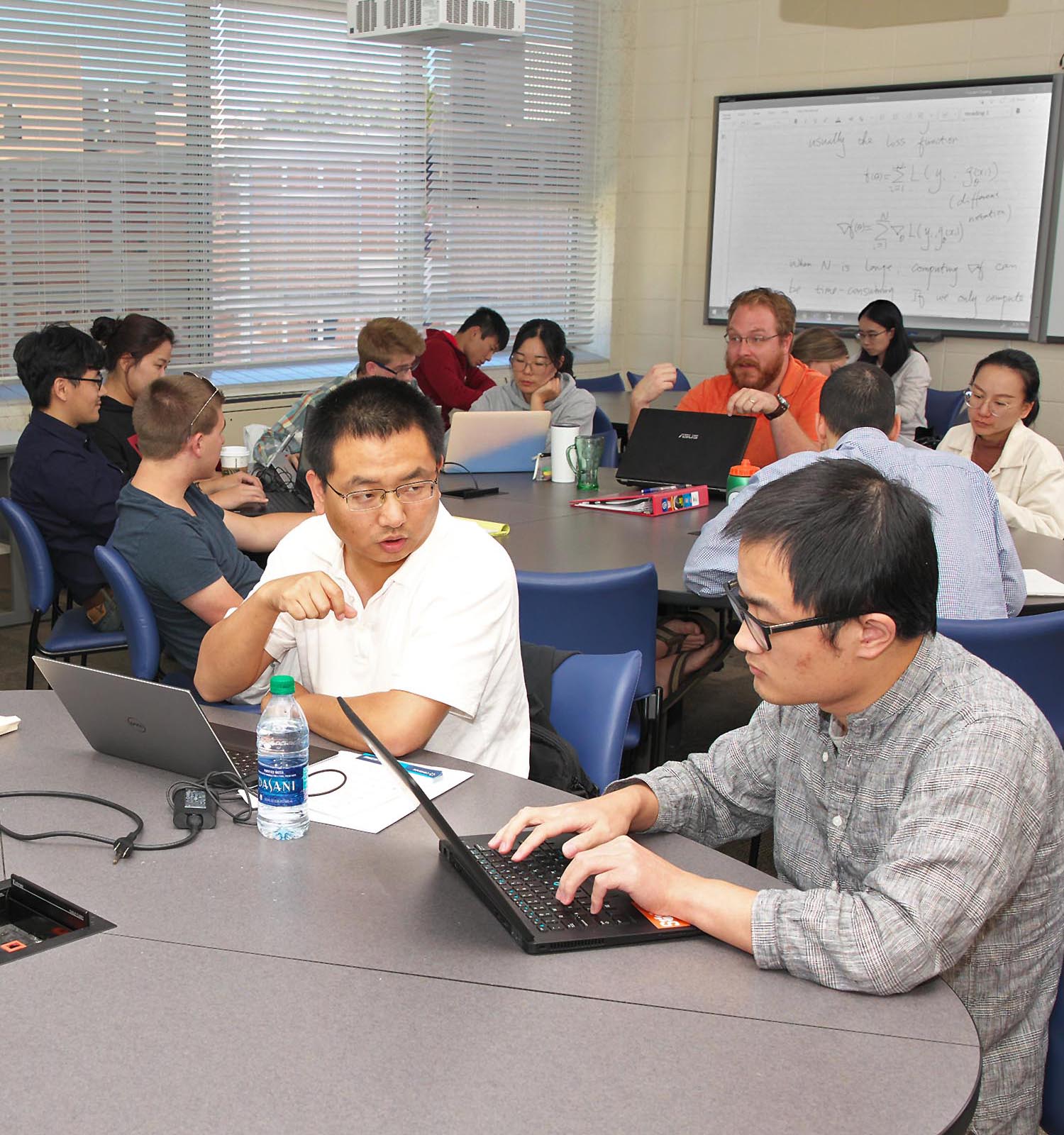
Programs of Study
Undergraduate
Undergraduate students in the School of Mathematical and Statistical Sciences can choose a B.A. or B.S. degree. The Bachelor of Arts option requires a few more liberal arts classes and includes a minor; secondary education math majors often follow this program. In the Bachelor of Science program, students can choose among several focused coursework options.
Master’s
The Master of Science in mathematical sciences program prepares the next generation of mathematical scientists. M.S. students will familiarize themselves with a broad base of mathematical techniques from many areas of mathematics. Students are involved in significant research projects, written publications and oral presentations. A Master of Science in Data Science and Analytics program is also offered.
Doctoral
The Ph.D. in mathematical sciences program prepares and trains experts and leaders in the mathematical sciences. Ph.D. graduates will be experts in their research fields, be familiar and competent in a broad array of mathematical sciences techniques, and will be effective communicators and teachers of basic mathematical sciences who can mentor and lead future mathematical scientists.
Top News and Features
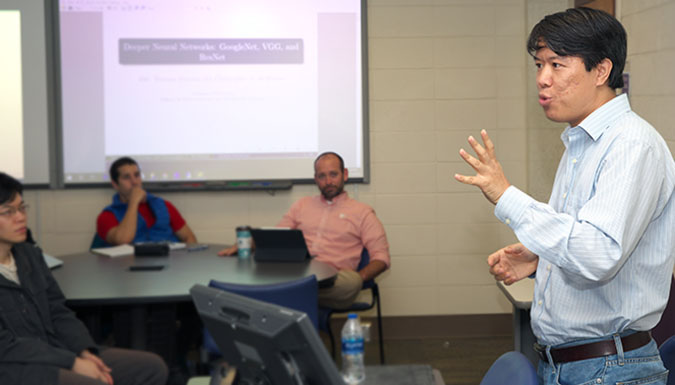
Rewarding Careers
Math-Related Jobs Expected to Grow 28% by 2030
The Bureau of Labor Statistics predicts that employment in math-related occupations will grow 28% from 2020 to 2030, much faster than the average for all occupations, and will add about 67,200 jobs. Today, you can find Clemson-trained mathematicians working for at U.S. and global corporations including Meta, Hubbell Inc., American Gaming Systems, Bank of America, Corning Inc., the Naval Information Warfare Center, and Equitable.
Explore Programs