Research Methodologies
Systematic Research
Through collaborations among the faculty members within the Department of Industrial Engineering, five research methodologies were formed out of the areas of specialization.
- Optimization
- Natural Decision Making and Resilience Engineering
- Statistical Modeling and Learning
- Stochastic Modeling and Systems
- Task Analysis
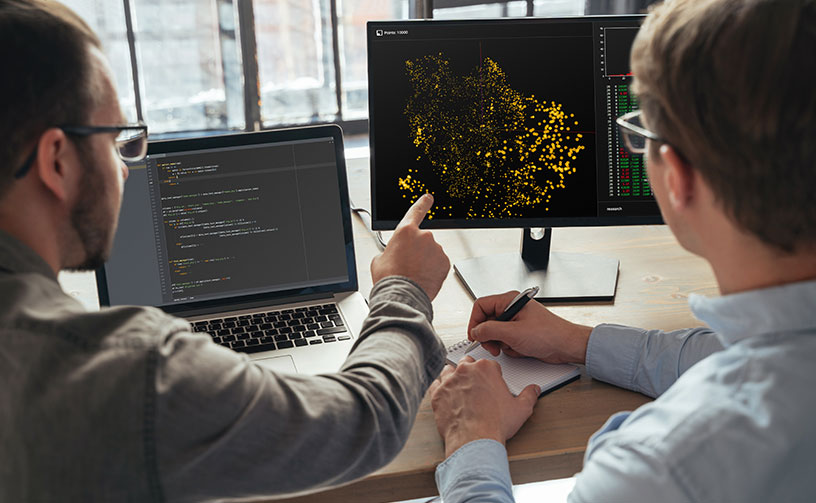
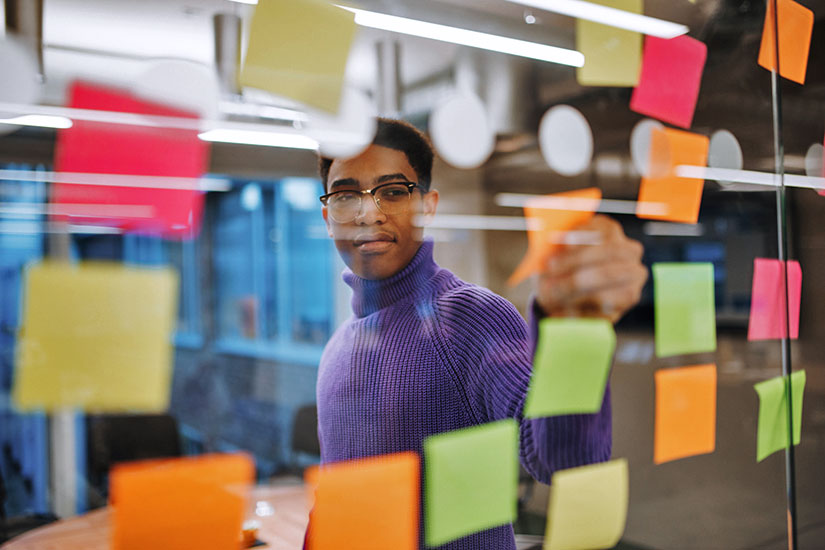
Optimization
Our methodological research in optimization spans theory, analysis, and design of computationally efficient, robust, and scalable algorithms to handle real-world problems in engineering, operations, economics, and business.
Optimization ResearchNaturalistic Decision Making
A key theme is identifying adaptive patterns and emergent properties of systems through proactive learning. Insights can be used to inform decisions at various organizational levels and in complex naturalistic environments in various domains such as healthcare, education, and crisis response.
Naturalistic Research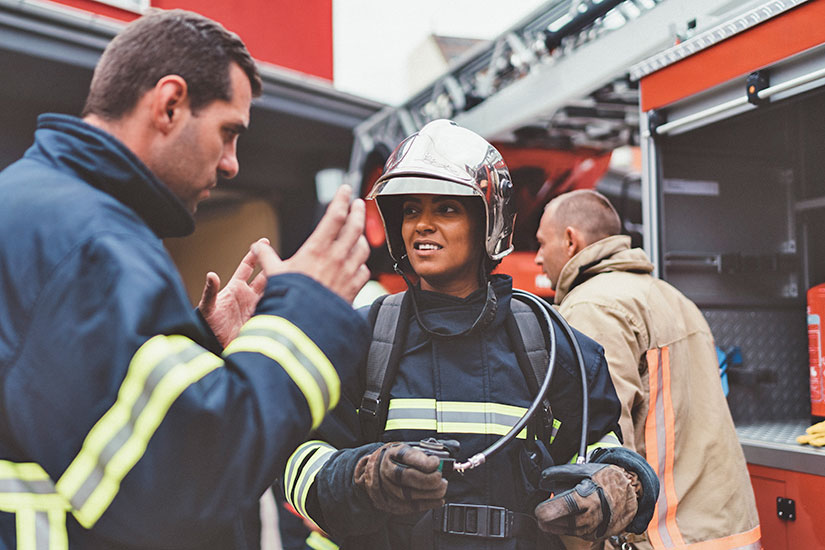
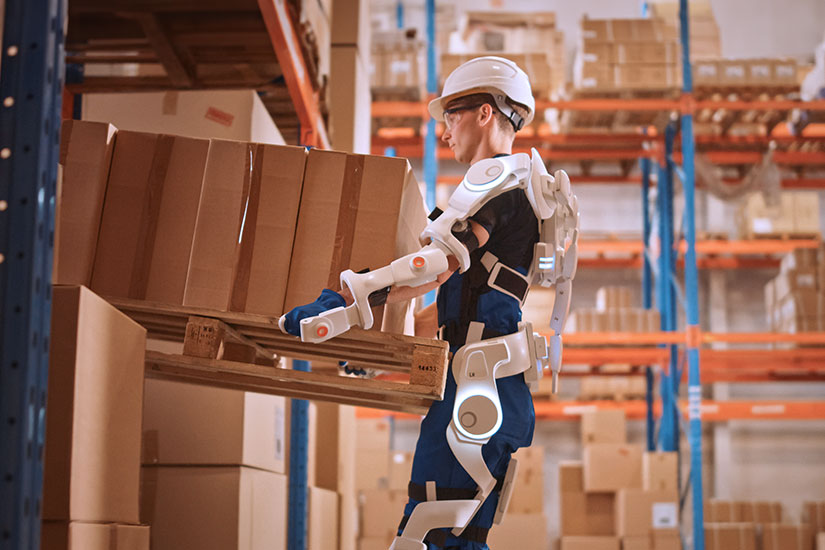
Statistical Modeling and Learning
Going from data to models, our methodological research is shaped around devising novel frameworks that can lead to fair and interpretable decisions and insights. Key drivers are the availability of data and computational powers in the last decade.
Statistical Modeling ResearchStochastic Modeling and Systems
Stochastic modeling is built upon probability theory, statistics, and stochastic processes to address uncertain, complex physical, cyber, and service systems. Our research spans a host of applications in supply chains and healthcare systems.
Stochastic Modeling Research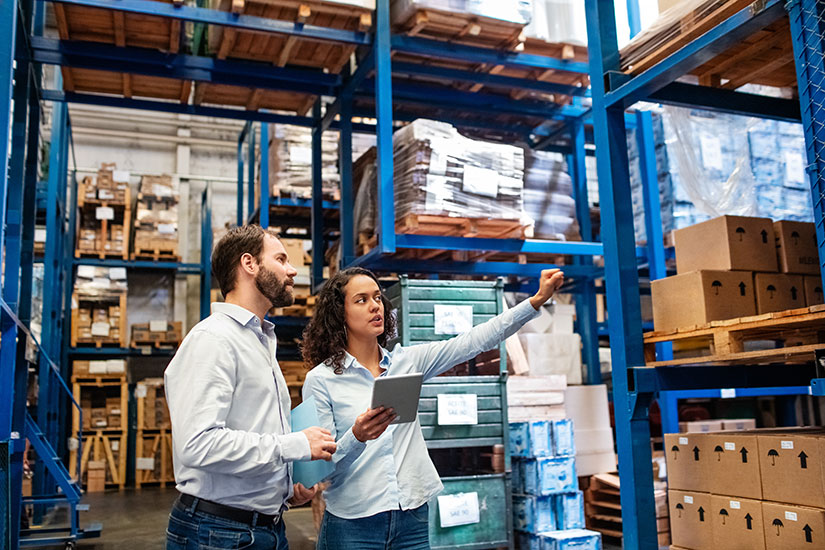
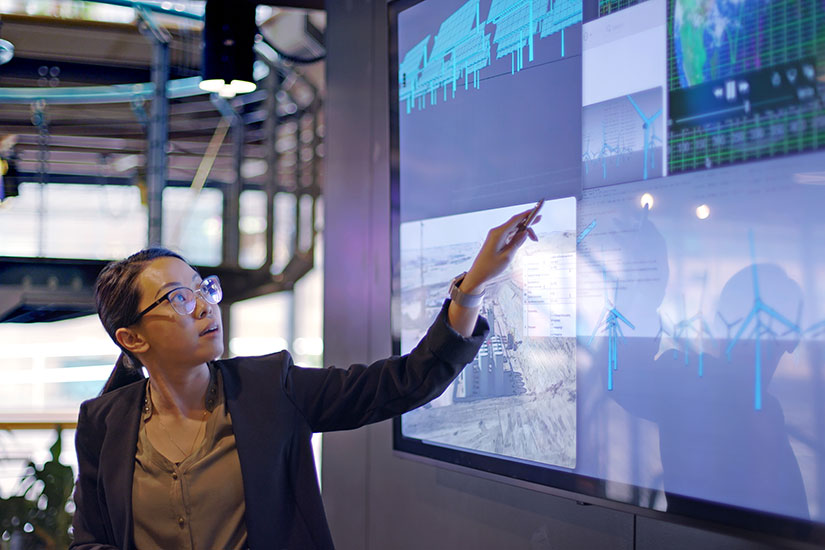
Task Analysis
The Department of Industrial Engineering is dedicated to gaining and sharing a better understanding of how tools, technologies, and work practices affect health and performance and how they can be improved through human-centered design.
Task Analysis Research